Agent-based modelling of a small-scale fishery in Corsica
- a Eric Innocenti ,
- b Corinne Idda,
- c Dominique Prunetti,
- d Pierre-Régis Gonsolin
- a,b,cUMR CNRS 6240 LISA, University of Corsica - Pasquale Paoli; Campus Mariani, Bât. Edmond Simeoni, BP52, 20250 Corte, France
- d Corsica Institute of Technology, University of Corsica - Pasquale Paoli; Campus Grimaldi, BP52, 20250 Corte, France
Cite as
Innocenti E., Idda C., Prunetti D., and Gonsolin P.R. (2022).,Agent-based modelling of a small-scale fishery in Corsica. Proceedings of the 10th International Workshop on Simulation for Energy, Sustainable Development & Environment (SESDE 2022). , 005 . DOI: https://doi.org/10.46354/i3m.2022.sesde.005
Abstract
In this work we introduce a new multi-stock, multi-fleet, multi-species and bioeconomic model for the complex system of a small-scale fishery. The objective is to study fisheries in order to ensure the renewal of the stock of biomass. This stock represents both a means of subsistence for fishermen but also contributes to food security. We model the system as a Multi-Agent System using both Cellular Automata Model (CAM) and Agent-Based Model (ABM) computational modelling approaches. CAM are used to describe the environment and the dynamics of resources. ABM are used to describe the behaviour of fishing activities. The main interest of the conceptual model lies in the proposed laws and in its capacity to organize hierarchically all the local interactions and transition rules within the simulated entities. We report preliminary results showing that our modelling approach facilitates software parameterization for the specific requirements implied by the context of a small-scale fishery. The main results of this work consist in the creation of a computer modelling structure CAM and ABM, which constitutes a preliminary for an optimized resources management. In a future development, we will improve the behavior of economic agents in order to consider the complexity of their decision making.
References
- Arthur, W. B. (2014). Complexity and the Economy. Oxford University Press
- Bailey, R. M., Carrella, E., Axtell, R., Burgess, M. G., Cabral, R. B., Drexler, M., Dorsett, C., Madsen, J. K., Merkl, A.,
and Saul, S. (2019). A computational approach to managing coupled human–environmental systems: the poseidon model of ocean fisheries. Sustainability Science, 14(2):259–275
- Banos, A., Lang, C., and Marilleau, N. (2015). Agent-Based Spatial Simulation with NetLogo Volume 1, volume 1. Elsevier
- Beauchemin, C. A. A., Liao, L. E., and Blahut, K. (2018). Tutorial on agent-based models in netlogo applied to immunology and virology. arXiv: Quantitative Methods.
- Benchekroun, H. (2008). Comparative dynamics in a productive asset oligopoly. Journal of Economic Theory, 138(1):237–261.
- Benchekroun, H. (2008). Comparative dynamics in a productive asset oligopoly. Journal of Economic Theory, 138(1):237–261.
- Benchekroun, H. and Van Long, N. (2002). Transboundary fishery: a differential game model. Economica,
69(274):207–221.
- Benchekroun, H. and Van Long, N. (2002). Transboundary fishery: a differential game model. Economica,
69(274):207–221.
- Bonabeau, E. (2002). Agent-based modeling: Methods and techniques for simulating human systems. Proceedings of the National Academy of Sciences, 99(suppl 3):7280– 7287.
- Bousquet, F. (1995). Les systèmes multi-agents et la modélisation de la pêche dans le delta central du niger: remarques sur une expérimentation. In Questions sur la dynamique de l’exploitation halieutique, Colloques et séminaires, Paris, Orstom, pages 141–166.
- Buck, E. M. and Yacktman, D. A. (2010). Les design patterns de Cocoa. Pearson Education France.
- Burgess, M. G., Carrella, E., Drexler, M., Axtell, R. L., Bailey, R. M., Watson, J. R., Cabral, R. B., Clemence, M., Costello, C., Dorsett, C., et al. (2020). Opportunities for agentbased modelling in human dimensions of fisheries. Fish
and Fisheries, 21(3):570–587.
- Buschmann, F., Meunier, R., Rohnert, H., Sommerlad, P., and Stal, M. (1996). Pattern-Oriented Software Architecture Volume 1: A System of Patterns. Wiley.
- Carrella, E., Bailey, R. M., and Madsen, J. K. (2019). Repeated discrete choices in geographical agent based
models with an application to fisheries. Environ. Model. Softw., 111:204–230
- Carrella, E., Saul, S., Marshall, K., Burgess, M. G., Cabral, R. B., Bailey, R. M., Dorsett, C., Drexler, M., Madsen, J. K., and Merkl, A. (2020). Simple adaptive rules describe fishing behaviour better than perfect rationality in the us west coast groundfish fishery. Ecological Economics, 169:106449
- Cenek, M. and Franklin, M. (2017). An adaptable agentbased model for guiding multi-species pacific salmon
fisheries management within a ses framework. Ecological Modelling, 360:132–149.
- Chen, Y., Li, X., Liu, X., Huang, H., and Ma, S. (2019). Simulating urban growth boundaries using a patch-based
cellular automaton with economic and ecological constraints. International Journal of Geographical Information Science, 33(1):55–80.
- Clark, Colin, W. (1990). The optimal management of renewable resources. Mathematical Bioeconomics, 2:–.
- Colombo, L. and Labrecciosa, P. (2018). Consumer surplusenhancing cooperation in a natural resource oligopoly.
Journal of Environmental Economics and Management, 92:185–193
- Dockner, E., Feichtinger, G., and Mehlmann, A. (1989). Noncooperative solutions for a differential game model of fishery. Journal of Economic Dynamics and Control, 13(1):1–20.
- Dockner, E. J., Jorgensen, S., Van Long, N., and Sorger, G. (2000). Differential games in economics and management science. Cambridge University Press.
- Dockner, E. J., Jorgensen, S., Van Long, N., and Sorger, G. (2000). Differential games in economics and management science. Cambridge University Press.
- Hanaki, N., Kirman, A., and Pezanis-Christou, P. (2018). Observational and reinforcement pattern-learning: An exploratory study. European Economic Review, 104:1–21.
- Hogeweg, P. (1988). Cellular automata as a paradigm for
- Hunt, J. (2016). Scala design patterns - Patterns for Practical Reuse and Design. Springer
- Idda, C., Innocenti, E., Prunetti, D., and Gonsolin, P.-R. (2020). Agent-based multicomponent spatial simulation of a fishery. In Proceedings of the 2020 Summer Simulation Conference, SummerSim ’20, pages xx–xx, San Diego, CA, USA. Society for Computer Simulation International
- Innocenti, E., Detotto, C., Idda, C., Parker, D. C., and Prunetti, D. (2020). An iterative process to construct an interdisciplinary abm using mr potatohead: an application to housing market models in touristic areas.
Ecological Complexity, 100882(44):1 – 9.
- Innocenti, E., Detotto, C., Idda, C., and Prunetti, D. (2019). Urban, agricultural and touristic land use patterns: combining spatial econometrics and abm/lucc. In 2019 4th World Conference on Complex Systems (WCCS), pages 1–6. IEEE
- Innocenti, E., Silvani, X., Muzy, A., and Hill, D. R. (2009). A software framework for fine grain parallelization of cellular models with openmp: Application to fire spread. Environmental Modelling & Software, 24(7):819 – 831
- Innocenti, E., Urbani, D., Bisgambiglia, P.-A., and Gonsolin, P.-R. (2016). A multicomponent modeling approach for fishery simulations. In International Conference on Agents, 2016 IEEE International Conference on,
pages 108–109
- Innocenti, E., Urbani, D., Bisgambiglia, P.-A., and Gonsolin, P.-R. (2016). A multicomponent modeling approach for fishery simulations. In International Conference on Agents, 2016 IEEE International Conference on,
pages 108–109
- Jørgensen, S. and Zaccour, G. (2007). Developments in differential game theory and numerical methods: economic and management applications. Computational Management Science, 4(2):159–181
- Le Manacha, F., Durab, D., Perecd, A., Riutorte, J.-J., Lejeunec, P., Santonif, M.-C., Culiolif, J.-M., and Paulyg,
D. (2011). Preliminary estimate of total marine fisheries catches in corsica. Fisheries Centre Research Reports,
19(3)
- Levhari, D. and Mirman, L. J. (1980). The great fish war: an example using a dynamic cournot-nash solution. The Bell Journal of Economics, pages 322–334.
- Lindkvist, E., Wijermans, N., Daw, T. M., Gonzalez-Mon, B., Giron-Nava, A., Johnson, A. F., van Putten, I., Basurto, X., and Schlüter, M. (2020). Navigating complexities: agent-based modeling to support research, governance, and management in small-scale fisheries. Frontiers in Marine Science, 6:733
- Long, N. V. (2011). Dynamic games in the economics of natural resources: a survey. Dynamic Games and Applications, 1(1):115–148.
- Long, N. V. (2011). Dynamic games in the economics of natural resources: a survey. Dynamic Games and Applications, 1(1):115–148.
- Rui, X., Hui, S., Yu, X., Zhang, G., and Wu, B. (2018). Forest fire spread simulation algorithm based on cellular
automata. Natural hazards, 91(1):309–319
- Sanchirico, J. N. and Wilen, J. E. (1999). Bioeconomics of spatial exploitation in a patchy environment. Journal of Environmental Economics and Management, 37(2):129–150
- Sanchirico, J. N. and Wilen, J. E. (1999). Bioeconomics of spatial exploitation in a patchy environment. Journal of Environmental Economics and Management, 37(2):129–150
- Schaefer, M. B. (1957). Some considerations of population dynamics and economics in relation to the management of the commercial marine fisheries. Journal of the Fisheries Board of Canada, 14(5):669–681.
- Schimit, P. H. (2021). A model based on cellular automata to estimate the social isolation impact on covid-
19 spreading in brazil. Computer methods and programs in biomedicine, 200:105832.
- Schimit, P. H. (2021). A model based on cellular automata to estimate the social isolation impact on covid-
19 spreading in brazil. Computer methods and programs in biomedicine, 200:105832.
- Sutton, R. S. and Barto, A. G. (2018). Reinforcement learning: An introduction. MIT press
- Tesfatsion, L. (2017). Modeling economic systems as locally-constructive sequential games. Journal of Economic Methodology, 24(4):384–409
- Tisue, S. and Wilensky, U. (2004). Netlogo: Design and implementation of a multi-agent modeling environment. In Proceedings of agent, volume 2004, pages 7–9. Springer Cham, Switzerland.
- Tuck, G. N. and Possingham, H. P. (2000). Marine protected areas for spatially structured exploited stocks.
Marine Ecology Progress Series, 192:89–101
- University of Corsica (2016). The moonfish project. https://moonfish.universita.corsica/?lang=en, Last
accessed on 2022-04-11.
- University of Corsica (2016). The moonfish project. https://moonfish.universita.corsica/?lang=en, Last accessed on 2022-04-11.
- Verhulst, P.-F. (1838). Notice sur la loi que la population suitdans son accroissement. Corresp. Math. Phys., 10:113–126.
- Wilensky, U. and Rand, W. (2015). An introduction to agentbased modeling: modeling natural, social, and engineered complex systems with NetLogo. MIT Press.
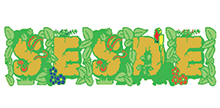
Volume Details
Volume Title
Proceedings of the 10th International Workshop on Simulation for Energy, Sustainable Development & Environment (SESDE 2022)
Conference Location and Date
Rome, Italy
September 19-21, 2022
Conference ISSN
2724-0061
Volume ISBN
978-88-85741-82-9
Volume Editors
Agostino G. Bruzzone
MITIM-DIME, University of Genoa, Italy
Janos Sebestyen Janosy
Centre for Energy Research Hungarian Academy of Sciences, Hungary
Letizia Nicoletti
CAL-TEK S.r.l., Italy
Gregory Zacharewicz
École des mines d'Alès, France
Massimiliano Schiraldi
University of Rome Tor Vergata, Italy
SESDE 2022 Board
Janos Sebestyen Janosy
General Co-Chair
Centre for Energy Research Hungarian Academy of Sciences, Hungary
Gregory Zacharewicz
General Co-Chair
IMS Université Bordeaux, France
Letizia Nicoletti
Program Co-Chair
CAL-TEK S.r.l., Italy
Massimiliano Schiraldi
Program Co-Chair
University of Rome Tor Vergata, Italy
Copyright
© 2022 The Authors. The articles are open access and distributed under the terms and conditions of the Creative Commons Attribution (CC BY-NC-ND) license.