UGV Digital Twin for Supporting Innovative Environmental Treatments of Large Areas by using AI
- a Antonio Giovannetti ,
- b Mehrnoosh Mashayekhizadeh,
- c Daniele Cefaliello,
- d Farshad Shamlu,
- e Hinda Taib
- a University of Genoa, Via Opera Pia 15, Genoa, 16145, Italy
- b,c,d,e Simulation Team, Via Cadorna 2, Savona, 17100, Italy
Cite as
Giovannetti A., Mashayekhizadeh M., Cefaliello D., Shamlu F., Taib H. (2022).,UGV Digital Twin for garbage detection through AI. Proceedings of the 10th International Workshop on Simulation for Energy, Sustainable Development & Environment (SESDE 2022). , 012 . DOI: https://doi.org/10.46354/i3m.2022.sesde.012
Abstract
The efforts to maintain low level of pollution in the environment need to meet sustainable and innovative solutions in order to be effective in the modern and complex world. Indeed, currently we are facing critical issues in terms of sustainability and new technologies are crucial to identify new solutions: recycling the abandoned wastes is a critical issue that needs to be considered and implemented beside their collection and processing. Due to these reasons, the purpose of this research is to develop an intelligent cleaning complex system based on UGV (Unmanned Ground Vehicle), which focus is to enhance the ability of strategic decisions making and achieve satisfactory trash detection in the wild for deployment. The system stands on AI (Artificial Intelligence) based on deep neural networks, which behaviour is simulated in a 3D Unity synthetic environment, through the usage of a digital twin, that simulates the desired solution of detecting and collecting garbage. The developed virtual environment chosen by the authors is a contaminated beach, in which an armed and autonomous robot manages to collect and recycle the wastes; the scenario is flexible to changes and further ones can be developed for training and testing of the intelligent system.
References
- A.G. Bruzzone et al. (2019a). Application of blockchain in interoperable simulation for strategic decision making. Summer Simulation Conference (pp. 1-10).
- A.G. Bruzzone et al. (2019b). A digital twin approach to develop a new autonomous system able to operate in high temperature environment within industrial plants. Summer Simulation Conference (pp. 22-24)
- A.G.Bruzzone et al. (2020). Modeling Human Physiology Coupled with Hyperbaric Plant Simulation for Oil and Gas. International Journal of Privacy and Health Information Management (IJPHIM), 8(1), 1-12.
- L.-C Chen, Z., P., S. and A. (2018). Encoder-decoder with atrous separable convolution for semantic image segmentation. Proceedings of the European Conference on Computer Vision (ECCV)
- G.-R Jocher et al. (2020). yolov5. Code repository
- Q. Lei et al. (2021). Garbage Classification System with YOLOV5 Based on Image Recognition. Intelligent Robot & Equipment Center Guangzhou Institute of Advanced Technology, Chinese Academy of Sciences
- Currie, C. S., Fowler, J. W., Kotiadis, K [Kathy], Monks, T., Onggo, B. S., Robertson, D. A., & Tako, A. A. (2020). How simulation modelling can help reduce the impact of COVID-19. Journal of Simulation, 14(2), 83–97.
- S. Majchrowska et al. (2022). Deep learning-based waste detection in natural and urban environments. Waste Management, Volume 128
- L. Parker. (2018). Here’s how much plastic trash is littering the Earth. National Geographic.
- U. S. Environmental Protecting Agency (EPA). (2020). EPA’s Report on the Environment (ROE). Human Exposure & Health: Disease and Conditions
- W. Zeng, R.L. Church. (2007). Finding shortest paths on real road networks: the case of A*. Department of Geography, University of California
- Z. Wu et all. (2021). Using YOLOv5 for Garbage Classification. 2021 4th International Conference on Pattern Recognition and Artificial Intelligence (PRAI)
- A.G. Bruzzone et al. (2014). Simulation exploration experience: providing effective surveillance and defense for a moon base against threats from outer space. 18th International Symposium on Distributed Simulation and Real Time Applications (pp. 121-126). IEEE/ACM.
- A.G. Bruzzone et al. (2016). Autonomous Systems for operations in critical environments. Simulation Series, 48(5) 17-24
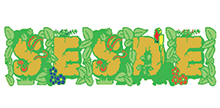
Volume Details
Volume Title
Proceedings of the 10th International Workshop on Simulation for Energy, Sustainable Development & Environment (SESDE 2022)
Conference Location and Date
Rome, Italy
September 19-21, 2022
Conference ISSN
2724-0061
Volume ISBN
978-88-85741-82-9
Volume Editors
Agostino G. Bruzzone
MITIM-DIME, University of Genoa, Italy
Janos Sebestyen Janosy
Centre for Energy Research Hungarian Academy of Sciences, Hungary
Letizia Nicoletti
CAL-TEK S.r.l., Italy
Gregory Zacharewicz
École des mines d'Alès, France
Massimiliano Schiraldi
University of Rome Tor Vergata, Italy
SESDE 2022 Board
Janos Sebestyen Janosy
General Co-Chair
Centre for Energy Research Hungarian Academy of Sciences, Hungary
Gregory Zacharewicz
General Co-Chair
IMS Université Bordeaux, France
Letizia Nicoletti
Program Co-Chair
CAL-TEK S.r.l., Italy
Massimiliano Schiraldi
Program Co-Chair
University of Rome Tor Vergata, Italy
Copyright
© 2022 The Authors. The articles are open access and distributed under the terms and conditions of the Creative Commons Attribution (CC BY-NC-ND) license.